The full form of ACF is the Auto-Correlation Function, a statistical measure that quantifies the degree of correlation between a time series and lagged versions of itself. The ACF helps us understand how well a time series is correlated with its past values and can be used to identify patterns and trends in data.
ACF full form in Hindi stands for स्वरूपित सम्बन्ध फलन (svaroopit sambandh falan).
Here are some applications of the ACF
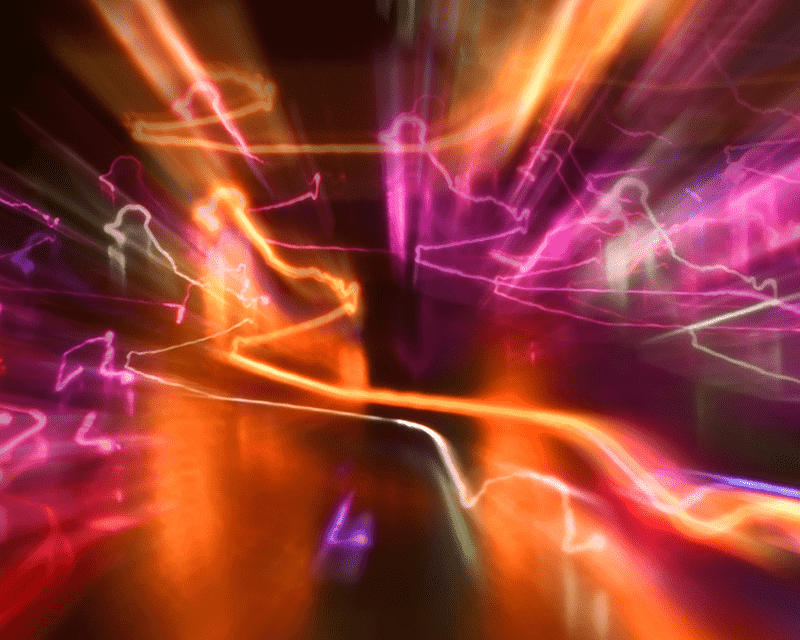
Identifying the order of an autoregressive (AR) model: The ACF can be used to identify the order of an autoregressive model, which is a type of statistical model used to describe the time-dependent behaviour of a time series.
Detecting seasonality: The ACF can be used to detect seasonal patterns in a time series by identifying significant autocorrelations at specific lag intervals.
Identifying the order of a moving average (MA) model: The ACF can be used to identify the order of a moving average model, which is a type of statistical model used to describe the time-dependent behaviour of a time series.
Explain Identifying the order of an autoregressive (AR) model
An autoregressive (AR) model is a type of statistical model used to describe the time-dependent behaviour of a time series. It models a time series as a linear combination of its past values, with each past value being weighted by a coefficient. The order of an AR model refers to the number of past values that are used to predict the current value.
The ACF can be used to identify an AR model’s order by examining the autocorrelations’ strength and pattern at different lag intervals. If there is a significant autocorrelation at a particular lag interval, it suggests that the time series is influenced by its past values at that interval. The order of the AR model is the highest lag interval at which there is a significant autocorrelation.
For example, consider a time series with the following ACF plot:
Lag interval: 1 2 3 4 5
Autocorrelation: 0.5 0.3 0.2 0.1 0.05
In this case, the autocorrelation is significant at lag intervals 1 and 2 but not at lag intervals 3, 4, and 5. This suggests that the order of the AR model is 2 because the time series is influenced by its past values at lag intervals 1 and 2.
It’s important to note that the ACF is just one tool that can be used to identify the order of an AR model. Other methods, such as the partial autocorrelation function (PACF), may also be used to determine the order of an AR model.
Explain Detecting seasonality
Seasonality refers to periodic patterns in time series data that recur at regular intervals, such as daily, weekly, or yearly. The ACF can be used to detect seasonality in a time series by examining the strength and pattern of the autocorrelations at different lag intervals.
If there is a significant autocorrelation at a particular lag interval, it suggests that the time series is influenced by its past values at that interval. If the lag interval corresponds to a known periodic interval, such as a week or a month, it may indicate the presence of seasonality in the time series.
For example, consider a time series with daily data. If the ACF plot shows significant autocorrelations at lag intervals of 7, 14, 21, and so on, it may indicate the presence of weekly seasonality in the time series. Similarly, if the ACF plot shows significant autocorrelations at lag intervals of 30, 60, 90, and so on, it may indicate the presence of monthly seasonality in the time series.
It’s important to note that the ACF is just one tool that can be used to detect seasonality in a time series. Other methods, such as decomposition or the spectral density function, may also be used to identify periodic patterns in time series data.
Explain Identifying the order of a moving average (MA) model:
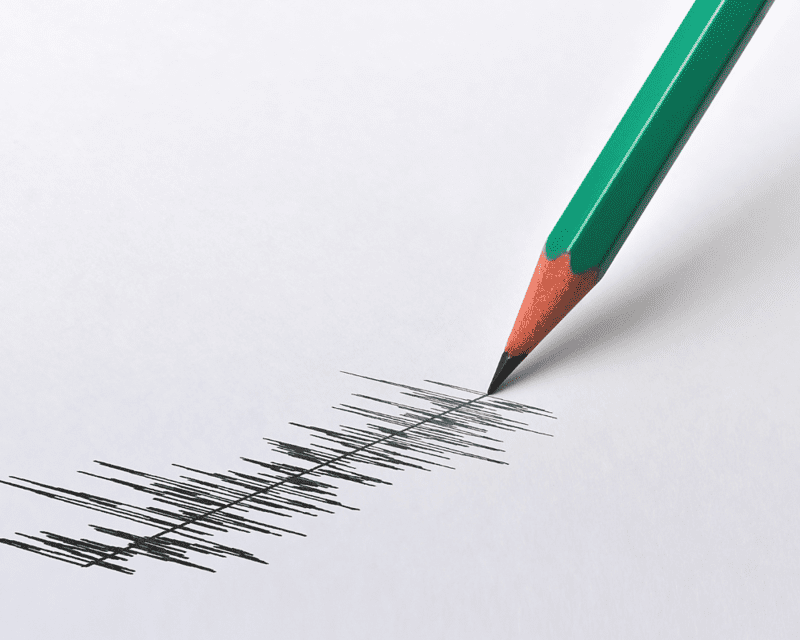
A moving average (MA) model is a type of statistical model used to describe the time-dependent behaviour of a time series. It models a time series as the sum of its past errors, with each error being weighted by a coefficient. The order of an MA model refers to the number of past errors that are used to predict the current value.
The ACF can be used to identify an MA model’s order by examining the autocorrelations’ strength and pattern at different lag intervals. If there is a significant autocorrelation at a particular lag interval, it suggests that the time series is influenced by its past errors at that interval. The order of the MA model is the highest lag interval at which there is a significant autocorrelation.
For example, consider a time series with the following ACF plot:
Lag interval: 1 2 3 4 5
Autocorrelation: 0.5 0.3 0.2 0.1 0.05
In this case, the autocorrelation is significant at lag intervals 1 and 2 but not at lag intervals 3, 4, and 5. This suggests that the order of the MA model is 2, because the time series is influenced by its past errors at lag intervals 1 and 2.
It’s important to note that the ACF is just one tool that can be used to identify the order of an MA model. Other methods, such as the partial autocorrelation function (PACF), may also be used to determine the order of an MA model.
Here are the steps for calculating the ACF
Define the lag interval: The lag interval is the number of time units the time series is shifted by to calculate the ACF.
Calculate the autocovariance for each lag interval: The autocovariance is a measure of the degree of association between a time series and lagged versions of itself.
Normalize the autocovariance by the time series variance: This step converts the autocovariance to autocorrelation, a standardised correlation measure.
Plot the autocorrelation values: The ACF is often plotted as a lag interval function to visualise the correlations’ strength and pattern.